Panel Discussion — Fluorescence Correlation Spectroscopy (FCS) Technology Focus
This is a machine transcription and therefore it may contain inaccuracies, errors, or mispronunciations. Notice an error you think needs changing? Please contact the Bitesize Bio team using this form: https://bit.ly/bsbtranscriptions
Welcome to the Microscopists,
a bite-sized bio podcast hosted by Peter Oto,
sponsored by Zeiss Microscopy. Today on the Microscopists,
Today on the Microscopist,
we have a special technology focus episode featuring developers,
experts,
and end users of something called fluorescence correlation spectroscopy.
Coming up, talk to Roland,
highlights how incredibly rich f c s data can be.
The movement of the, of these molecules contains so much information.
It contains information about the environment, right?
In what kind of environment are you more viscous, less viscous?
It tells you something about whether these molecules interact with something.
If you interact with something, you move slower. If you, uh,
it tells you something about how you move, right? If you have flow,
you get a different kind of, uh, signal. Then if you randomly move around,
right?
Annette er from Zeiss Research Microscopy Solutions highlights how
airways scan technology is being adapted to make f c s more user-friendly.
In this case, this was really a very
circular way to approach this because since f c s is not so in focus,
it's maybe not as straightforward as increased speed or increased resolution or
increased sensitivity. What is in microscope be obvious? So we,
it came together, um,
the area scan technology with its benefits for F C s and the idea to make
something very approachable for everybody.
And Chris McDonald here from York with me,
shares how much simpler Dynamics Profiler makes acquiring data on
intracellular dynamics.
And if you look at her data and my data, they're remarkably similar. So again,
I was, you know, going into it with,
with nothing to no expectations,
but actually being genuinely quite impressed with the results we got
All in this episode of The Microscopist.
Hi, I'm Peter Oto from the University of York, and welcome to today's special,
uh, podcast with the Microscopist,
which is actually a technology focus one talking about fluorescence,
correlation spectroscopy and dynamics profiler. And today as our guest,
I have Torston Rollan from the National Institute, uh,
university of Singapore. Morning Afternoon. How are you today? Torston?
Hi, how are you?
No, I'm, I'm, I'm very happy to be on the Post, uh, podcast.
Thanks a lot for the invitation.
Yeah, no problems. I've got, uh, Annette, about from Zeiss.
Hello.
Uh, based in Yna.
Yes, based in Yna, living in Yna,
I in Germany. And I have Chris McDonald,
actually from very local to me from University of New York at York. Chris,
how are you?
I'm doing pretty well, thanks.
So this is slightly different. I have a,
we have three individuals here have a very different take, uh,
or vested interest, maybe should I say, on the technology, uh,
Tossin as a technologist.
An Etta is the one who's actually hopefully making it more user friendly and
Chris, uh, as an end user perspective to,
to give us a wraparound of why,
why we are interested in this, what it can do for us,
and the importance in general to science and what it can do for us in the
future. So actually, I'm gonna come to you first Torsten, if that's okay.
And maybe just introduce your background yourself a little bit and why you are
interested in, for essence, correlation spectroscopy,
which I think from here on we'll call F C s.
Yeah. So I'm, I'm actually a physicist, and I started, of course,
like every physicist, uh, wants to do astronomy.
And then I realized at some point, um, um, I, let's put it that way,
I met the right person, um, uh, who showed me, uh, something about biology,
and I got more interested in biology and biophysics.
And so I started actually this career in biophysics and in fluorescence.
And then I started, uh,
working my PhD then in fluorescence collation spectroscopy.
And I never really left the field, so I'm now joined here, the,
the Department of Biological Science in, in, in Singapore. Sorry.
No regrets. Not carrying, gone into the stars.
Well, well, as a physicist, you always regret it a little bit. No, no, no. Not,
not really. I'm, I've been very, very happy, uh, uh, with career up to now.
And I, and f c s is a fantastic technique,
and there's always something new to do. There's always something, uh,
to improve. And, uh, that's what we've done over the last years, I hope,
Which I, which I think is amazing. I, we, we'll,
we'll talk about the latest developments in F C Ss. I,
I remember hearing the first time I was exposed to f c s was think in 2002,
that's a embo course at E M B L. Uh, and Petra Sch Willer, uh,
was one of the speakers. And actually,
Petra Willer got HandsOn in the demonstration. And it wasn't just f c s,
it was interesting.
I learned so much about G F P and blinking and the phenomenon and concentrations
and everything else I've not had to think about before that actually,
just learning around the technology helped to inform a lot of other strategies
as well. It's amazing. It's still, that's 20 years ago, 21 years ago,
and it's still developing. That's nuts.
Yeah, it is. I, I mean, the, the biggest thing about this is really, you know,
20, 30 years ago when we started, right? Uh,
you needed to have a custom build system,
and you needed to do all your data treatment, uh, alone. By now,
technology has developed so much that basically with almost all instruments we
have, you can do actually F C Ss. Most people just don't know it or don't do it,
but they should,
Which we'll hear more about in a moment, that, Annette, if I can come to you,
please. Mm-hmm. What, what's your expertise in this, you know,
how did you get into Life Sciences and F C S O?
Okay. Um, so I am, by training, I'm a developmental biologist.
I did a lot of, um, microscopy, uh, but more imaging based.
And then I joined Zeiss 15 years ago, roughly. And, um,
I'm now responsible for the L ss m in the mar as
a marketing manager. I just think a little bit about my job title,
I have to say, um, and switching them, uh, uh, quite a bit during my time.
Um, and so I have to say,
I'm not at all an expert in F C s,
so my role is very much looking into the
applications that are needed, what is developing. And so I,
I normally get into topics in a very short amount of time and kind of
zoom into them and, uh,
try to understand them more from a kind of broader perspective.
But I will not be the person who can explain to you every mathematical formula
or effect in detail, I would say. I, I think that's fine.
Um, that's,
You say your marketing manager?
Yes.
Okay. So my interactions with you have never been about marketing particularly,
but very much been around the development of new technology. So,
Right.
How, how closely is that entangled marketing manager?
Doesn't sound like your role from my, in my interactions with you in the past.
Okay. Um, I can see how this might be, um,
maybe a bit confusing, but it is very close. I mean, it's not when,
when you think of marketing in,
in our field of work microscopy where you are not,
let's say selling a lifestyle or an idea like, uh, maybe a soft drink would do,
but you are focusing on what is really needed in the field and where the
scientific focus is and where people are heading towards.
So to improve their everybody's life, you have to look in, in,
in terms of marketing manager, you are really looking into the market, um,
looking what's trending, looking, what's maybe beneficial. And, um,
then you are very close in making decisions or being part of
the decision, what will be developed next and how this should look like,
which kind of technology will be used.
And then during this process of development, um,
we are extremely close to our product managers. And, um,
we have to be close to development because even though we might not become
experts, we need really to understand the pros and cons, um,
that can do and can't do of everything we are doing.
So then we cannot make promises that are faults,
but can essentially kind of cook it down for everybody to understand.
And that's where it gets very heterogeneous. And, um,
maybe next time we speak, I have to point out more about marketing material, so
I'm not sure
I, I know where, and so, so actually here at York, we do a lot of, uh,
beach testing for ice. Yeah. And I,
I do appreciate we need a lot of,
not just necessarily the scientific scientifically best data,
sometimes it needs to be marketing data, which is subtly different in it.
I I do know that you, you are asking for that as well, but the,
I think what was interesting, we need a podcast actually on careers, because I,
I think that what you've just described is something very different to what most
people, or certainly what I would assume mm-hmm.
Will be a marketing manager's post. I,
I'd be thinking it's all about how to promote it, how to do the social media,
whereas actually you are looking at the content, right? The, the market is,
it's very close to a product manager and the application side, it's,
it's easier, you say, become very mixed up with those. It's,
It's,
it's a really heterogeneous mix here because you're looking as well at business
opportunities. You're kind of at this intersection of technology,
application, business, and then marketing.
I do work with our marketing specialists with, um, the messaging, the wording,
the campaigns,
because you need to understand the technology and the benefit to then
do the wording, right? So this is really, this, this way it becomes, um,
very interesting, I would say. And exciting.
Okay. Thanks. We'll come back 'cause you've got a, a, a business need.
And I'll, I will come back to, uh, that obviously in a bit. And Chris, uh,
you, what, what is your active research in at the moment?
And did you always want to be doing what you're doing now?
I would say since I was in undergrad, when I first did a lab project,
then I decided to get into research, but I,
I had no intentions of doing a PhD until the very end of undergrad,
to be honest. Uh,
and then since then we've been working on membrane, well,
I've been working on membrane trafficking throughout my entire career,
so PhD postdoc and the stuff we've been doing since I came to York,
and like, it's fundamentals, I suppose.
Membrane trafficking is a movement of proteins, lipids throughout a cell.
So this lends itself to things like microscopy and cent proteins,
you know, so there's, we tag things and we watch them and we,
uh, we do a lot of work with yeast. So budding yeast,
there's powerful genetic tools.
So we can break pathways by deleting genes and then seeing the consequences that
has by microscopy.
And, um, so you are using yeast, I presume, as a model system. Uh, a again,
if I go back, if you pull nurse as a prime example of this at cancer research,
Orrick, uh, with a cancer research using yeast as a model system,
I presume you are, you, you're not so interested maybe in the yeast per se,
but using it as a model system?
Yeah. Uh, so different yeast from poly nurse, we use budding yeast.
He uses fission yeast, but the, the, the idea is the same. We,
the things we study are highly conserved across all
ocs. So we can do genetic screens and yeast, but the things we focus on that,
you know,
human cells have counterparts that we think perform in the same or similar ways.
So yeast as a model, you know,
it's a tractable model for conserved processes across
thetic cells.
Okay. So, so you're be interested in the,
the dynamics of these proteins are trafficking sites. So actually,
if I come back to you, to the dynamics of proteins, uh,
lipids and proteins both are saying that where you've actually made your career,
uh,
an expertise in with the different biophysical techniques that
you've been at, the pioneering engage.
So can you describe a bit about what F C s actually does and how it does it?
Ah, yeah. Okay. So, um, f c s in principle, uh,
analyzes fluctuations, right? And tries to get information out of there.
So how do you best imagine that? So let's assume you live in a city,
okay? You open your window, so what you gonna hear?
So lots of noise, car noise,
Ex Exactly. So now you're gonna hear car from time to time, right?
Um, so how do you analyze that? So, so first of all, it just sounds like noise.
You don't think, uh, think about it. But if you, if you, if you listen to it,
you hear like, well, well, well, right,
so this actually contains information.
It contains information about how many cars they are.
It tells you how fast they are, right?
Depending on whether you are live at a highway or live in a city, right?
Cars go with a hundred kilometers per hour, 30 kilometers per hour. That will,
you'll hear that.
And you basically can analyze that by averaging all these, uh,
all that information basically statistically evaluated.
And then somehow the statistics will tell you there were so many cars on
average, and they moved with that speed on average. Now,
if you replace cars with fluorescent molecules and hearing, we seeing,
we at f c s, so what we do is we basically look at molecules coming by,
and each time they come by, they give you a fluorescent peak.
And from these peaks, you can evaluate how fast these molecules move.
And the interesting thing is, the, the movement of the,
these molecules contain so much information,
it contains information about the environment, right? Uh,
in what kind of environment are you more viscous, less viscous?
It tells you something about whether these molecules interact with something.
If you interact with something, you move slower. If you, uh,
it tells you something about how you move, right? If you have flow,
you get a different kind of, uh, signal than if you randomly move around, right?
And that is what FCS can, can get for you.
So it measures on one side concentrations and diffusion,
but it can extract so much more information than about the actual cellular, uh,
environment and its interactions.
So I, I, I've gotta say, I love the analogy of opening the, the,
the window in the city and listening to cars.
I've not heard that analogy before and know,
I'm going to be using that analogy because you can visualize it here, whatever,
picture it in your head really, really clearly. So this is,
this sounds great. Uh, and you've explained the viscosity, the interactions,
and these are very important for all sorts of cell signaling. You know,
take it back to your medicines, your drug designs, everything else, uh,
underlying disease, these interactions,
viscosities are very important to understand.
So we can understand how to treat or what, what's causing them to start with.
But if it's so good, I, I, and I, I,
I've gotta be careful not to make this aquis. And it, I, we,
we have F c s and F C C S at York,
but only a few users really embrace it and take off with it. Why,
why, why isn't everyone doing this then?
Yeah, that's a very good question. No, the, the simple thing is that the,
the problem lies in the evaluation of the data, right? As I said, I mean,
you evaluate the data, you get a statistical,
that's what we call the correlation function, the auto correlation function.
And that needs to be fitted and interpreted. And that, uh,
at the moment is mostly done by expert users. They're looking at that,
they're trying to fit the data and to have an intuition for,
for the function itself takes some time. So it is,
it is at the moment, not necessarily a push button technique. However,
that I think with the advances in technology we have right now,
we can get there. And I think that shouldn't take too long before we, at the,
at a level where we can do f c s measurements and, uh,
you get the data out without having an expert user around you. I mean,
I think that's the ultimate goal for us.
Yeah, I, I, I think you've, that,
that's certainly my experience as well with it, that it's, I, I, you know,
the users that we use it, I,
I get quite excited when the user says they're going to do f c s. Uh, I,
I get my team now to help the user, but I get involved.
'cause I've got to say they're more competent.
My staff are more competent on the system than I am in some cases.
And certainly for the F C s, they're certainly more competent in F C C S,
but actually I'm a default wrapper. So I,
I would use frap to look at protein dynamics,
which is great when you have a high concentration.
But also F C SS hasn't historically been able to cope with the high densities of
some of the proteins. Uh, certainly in our case.
Is that the case for yourself as well? Ster?
Um, in principle, yes, in principle, that's correct. Um,
the high concentrations can be a problem, but actually most of the, the,
the proteins we look at are never expressed at these high concentrations.
So typically when we look for, for proteins in a cell, most of the time,
if you take confocal microscopy, we can't even see the cell that are the cells.
We measure f c s in very often because actually the proteins,
we don't wanna overexpress the proteins.
If you of course have an overexpressed system,
or you have a system that is really very, very highly expressed,
then you have to, then again, there, there are tricks. I mean,
there's somebody who showed that you can go up to 40 micromolar concentrations
or also, right? Ted Lauren has done that very nicely, but, uh, you're right,
their fr might be the, the, the better option.
I, I think that's a a lovely point. You know,
so in some of these biological cases,
people are expressing a protein of interest, uh, which can, there, if it's,
if it's, especially if it's fluorescent proteins, at that point,
it may no longer be end do endogenously relevant. In other words,
you may have one. So my, my analogy now, uh,
if I'm giving you lectures and there's a hundred seats in the lecture theater,
that's a hundred binding sites for people to go and sit on.
If you now put 1000 people in the room,
900 are going to be walking around looking for a binding site looking very
dynamic. Whereas the 100 functional people that are sat down,
or maybe they were asleep and not functional, I dunno which way it would be,
would actually be the immobilized ones at a very different diffusion rate.
But you'd see the 900, not the 100. And I guess that's what you are suggesting,
that actually sometimes you shouldn't be looking at those high concentrations.
But actually I think this system will come, come two with dynamics profile,
which is the latest innovation in this area.
So that I've found quite useful is being able to look at the concentration and
then comparing concentrations with the diffusion rates to show that
you are not in a, an odd well,
and that you are seeing linearity,
that that relates to the concentration and the diffusion rates in the
properties. And I presume you've been looking at that anyway.
So the nice thing about F C Ss,
you can get the concentration of your protein or your lipids as well, sort of,
Uh, yes, you can. I mean, but I said most of the time, uh, for us,
uh, we, we, we try to, uh, work at endogenous levels. So we,
we have actually, typically concentration are very, uh, relatively low. And, uh,
with lipids, same thing.
We typically label with a very small amount to disturb the system as little as
possible. But each time you put a label on something, you,
you disturb it in some way. Yeah, yeah.
So especially biologists are very tough on, on, on f c s, uh,
experts on that one. I'll come back. So, oh no, you can't label so much. You,
you, you, you know, it's not, not relevant anymore.
Yeah.
But then everyone using fluorescent protein has inevitably added a tag,
and, and it's the best tool we have at the moment. So I'll defend, uh, I have,
I'm a fluorescent microscopist. We have to defend tags at this point. Uh,
I can I ask you a question? Please. That, you know, this sounds wonderful.
So why, why did we need new innovation? What,
what were the limitations that you saw that you've helped to overcome?
Uh, ooh. So how do I approach this question? Oh, that's, that's the question,
right? So how, where do I start? Um, I think one is, um, that, um,
f c s is, um, or was a kind of, uh,
an expert method, right? So that was something that was very, um,
I was at least very aware of it because I would get a training and then I had a
break,
and then I would need to get trained again to learn all the buttons and twists
and so on and so forth. It's maybe a little bit my background. Um,
so why does it need innovation? I think one was that we said, okay, what,
when we go into planning what we do next,
maybe I kind of c can I circle your question a little bit? Um, yeah, of course.
Do, yes. So, because it's d because when we, when we plan what we do next,
I mean, we do look from very different perspectives, right? So what,
what I said what's trending? What is needed? Where is, where are you heading?
Um, on the other hand you look, what do you have, um, what is,
what is being developed? What kind of ideas are there in terms of technology?
So we speak, uh, with people who, so we are looking in all those directions.
And so maybe as there is sort of an analogy, if you're planning a new feature,
you would say, okay, um,
if I have a kind of plate of wood and four sticks,
I might make a table, right? That's very logical.
And then this is fantastic 'cause the technology would give you this,
but if you already have 10 tables or nobody needs a table because you are
sitting on the floor eating, then this is a not the way to go.
So you might make a shelf or something else.
And this is what happens in those cases. You look what you have at hand,
what kind of innovation there is, and then you see is does it even make sense?
And what kind of vision could you have for this, for your users? And, um,
in this case,
this was really a very circular way to approach this because since
f c s is not so in focus,
it's maybe not as straightforward as increased speed or increased resolution,
or increased sensitivity. What is in microscopy obvious? So we,
it came together, um,
the area scan technology with its benefits for F C s and the idea to make
something very approachable for everybody. And my vision together with,
um, dear colleagues of mine was at the beginning to say,
let's take this and make this for everybody.
Like everybody who does a live experiment can use it at the end.
And this came together, and I can't, I,
I remember pretty much the day and the moment where we wrote this down,
and from then on, you follow this kind of guiding star. But it's, it's very,
it's a very dynamic process until this comes together and then you move into
this direction and, um, yeah,
go along this way. And, and, um, maybe as a last sentence, um, in this process,
you get to know your feature better and better,
and then you kind of, it, it develops a very nice, in this case,
it leads a very nice dynamic and, um, yeah, it's vision.
Chris, just a heads up before I come to you,
everyone's had a really good analogy,
so I hope you're working on one at the moment.
I'm kind of conscious of that. Actually,
I was feeling a bit underprepared because they do not have an analogy.
I think these analogies are lovely. 'cause actually,
if you're not expert in the field,
it does help you understand the logic and the thought process and why these
things are important. Uh, I, I was, oh gosh,
now I've forgot my train of thought now. Uh,
so f c s has been really a more niche specialized because of that level
of expertise. Mm-hmm. Desires to do what they've done to try and make,
and we'll talk about the product, which makes it more user-friendly. Mm-hmm.
Part of that nicheness was expertise, but, you know, if you need it,
you'd have done it. I, Chris, you had to go with classic ffc s so you know,
when you need it, you would go for it. Mm-hmm. So where,
why did you think there was a market for this?
And it's not just something that just, you know, it's just, you know, the,
the technique for the, a niche technique that just Right,
people want what made you think or realize that actually this,
this is a technique that actually has a big audience,
just a big audience can't actually access it yet. Mm-hmm.
Mm-hmm. So I think it's when you, yeah,
when you think about it, um, you can measure more than the eye can see.
So even with normal super resolution,
you will not get to this level of information. And,
um, the idea was, um, with all those different data sets we have,
um, going into living samples, one is fundamentally missing.
So you can characterize yourselves in terms of the shape, the behavior,
maybe the, how they divide, how they arrange.
But the underlying dynamics are essentially cut off.
And that's exactly the point. You don't go there if you don't have to,
but who wouldn't go somewhere to explore something if you don't have the,
if, if you have the ability to just explore something?
I think that's something that is so much a scientific view, at least for me,
that if, if you open a door and you walk through it,
you never know what you might get.
So there might be a completely new way to characterize your sample if you're
able to measure concentration, even if it's,
even if it's not indigenous anymore.
So even if you are comparing your different mutant strains that you created and
wanna see, okay, which one is maybe the lowest concentration?
Or if you have had a very heterogeneous expression level,
I can now not look at this with intensities, but I can put numbers on it.
And, um, this, that we probably come later to it with,
we could even expand it a little bit.
But this was the initial idea that if you are exploring,
you can find things that you might have not known,
and you might realize it's actually something that I wanna explore more,
or wanna go down this path, even with other technology,
but you have to open this door. And, um,
so yeah, I, I think that's a very personal thing. So I did this,
this l s m in my studies,
I threw a dye on my little modern organism and I saw something and
somebody next to me said, oh, this has never been published. Nobody knows this.
It's very simple, right? It's not,
and this is the idea behind this that you say, oh, well,
you never know what you're gonna find. So why is there a market?
I think because it's so far, it's not been done enough.
I think it's really something that has been left out in the, in, in,
in this field of life imaging. And of course it's a bit, um,
it's a bit different than the other passes we went. There's a, um, there, uh,
there is some line of thought where we have to say, okay, we need to convince,
we have to do a little bit of convincing,
I guess, halfway through that net where you were talking about, you know, look,
going through new, new Frontiers.
I thought you were going very Star Trek on me for a moment. Kept pushing into,
I have to admit, I'm a, I'm a very trekky person, but that's a different story,
I guess.
Oh, so, so were you a Star Trek or Star Wars person that's in Star Trek? Stark.
You're Star Trek? Mm-hmm. Chris Star Trek, star Wars?
Neither,
Neither, or either.
Neither either.
Okay. And Torsten?
Probably a little bit more Star Wars actually.
More Star Wars. It
Was kind of the first movie I saw, I think in the, in the theater.
Wow.
Tells you how old I'm
Yeah, but I, but I, I,
I'm sure I saw James T. Kirk on TV before I went to see the movies.
Oh, that, that could be. But it was every week, so it was not a specialist,
this one movie.
That's better. I also, actually, just thinking about the Star Trek theme, uh, I,
I, I, Annette you sent one or two slides. Yeah.
And you've got the nice p s F over on my side here. Uh, and it just, you know,
it's like beam me up Scotty now, isn't it? You've got this Nice,
I'm expecting to be, so actually let,
let's maybe concentrate on the technique we've heard. Mm-hmm. Uh,
I dunno who wants to describe this, whether it's torsten or an net,
it's an net vigor, but obviously Torstin,
you are more than qualified to talk about this as well.
And over on the right we have the point bed function. So this,
I guess is the molecules moving in, moving out.
You can essentially think of it as a,
an hour shaped glass for those that are listing. Mm-hmm.
And you can see a particle moving in and a particle moving out. This is,
this is your cars coming into earshot and out of earshot.
Uh, right. So,
so it's interesting that this is just one point.
It's fairly static. Mm-hmm. Most Pierre for, for most techniques it's a,
a static point that you are, you are opening one window, Torsten, is that right?
Mostly?
Uh, that's that, that's right in principle. But you okay.
There the analogy breaks down. You could open multiple windows of, of course,
but each window has to be somehow separated from the others. Otherwise, uh,
you lose your, your fluctuations. Right. You can't hear anything anymore.
So the points, batch function tells you how,
how far the, the information for window can travel inside the house. Mm-hmm.
So it gets a bit complicated. Well,
Which is quite good. 'cause this is now starting to appreciate, and you,
to be fair, you've all made it sound really super easy at the moment. And,
and it's not the actual mass behind this, the, the,
the logic is actually not so easy. Mm-hmm. Uh, for the average biologist,
this is quite scary.
It's conceptually quite difficult sometimes to get your head ran,
how it actually comes out with a, a relevant biological number at the end.
Mm-hmm. So how do you, how, how do you make this approachable?
Like who answer maybe talk and go first.
Oh, okay. No, no, no. I, I I think that is, that is exactly the problem. I mean,
the calculation of this auto collation function,
which is very similar to a FOIA transform, is not super difficult. Right.
The problem is the interpretation. The problem is actually the fitting.
And like, how do you know how the auto correlation function should look like,
right? I mean, you get the function, it's like,
how do I know how to extract data from that?
That is actually the really hard part. And, but again, as I, I said earlier,
I think we are at a, at, at a,
at a stage where we can do this almost automatically.
So to, to, to remove actually this step from the, from, from, from,
from the normal user with quality controls, right? To provide the,
the data instead of having the,
the user actually sit there and trying to read a mask book about fitting data.
Mm-hmm.
And actually, I'm gonna come to Chris first. Chris,
this is F C Ss as you know it or knew it, uh, with single point.
And from recollection you did a bit of F C s before Dynamics Profiler.
Yeah.
So what you describe about biologists being scared of the maths and
you know, kinda knowing roughly what the software does, but not knowing how to,
to use it. That that's a good description of what we did previously. So,
I mean, we have collaborated with the physics department here at York.
So we've published various papers of Mark Leak and they've done, you know,
they have their custom built microscope and their custom built analysis and they
can get at some of these parameters for sure. But then,
you know, it,
it is more limited in that when we want to couple it to Mitral foot,
for example, which we can do with all the different hair scan two and LA Sim,
we were struggling to do that with that other system.
So then we did have a bash with F C Ss, again,
with help from Tes Stanley, from Ian Hitchcock's lab Yep.
And other people from the technology facility.
And then my memory is we were addressing reviewer comments
and we tried biochemistry fret and F C s,
and we just kinda needed one of them to work. And the fret worked really well,
really quickly. And then some of the biochemistry was making sense.
So when we were trying f c s, it was kind of complicated.
There was beads calibration, you know, it, it just,
it just didn't all come together. So I've been kind of aware of the,
the functionality of F C s, but we've never, you know, we've never published it.
We've never performed a full data set.
So that's why I was interested in this dynamic profiler,
because it sounded like an easier way to get at these parameters that,
you know, I mentioned we study membrane trafficking, surface membrane protein,
so transmembrane domain proteins in the plasma membrane.
So obviously we care about the environment and how they're,
these change in different conditions and so on, as,
as Thurston kinda described in the introduction.
Okay. So,
and maybe this is the best time to actually say what the innovation is,
what is different? So Dynamics profile, dp, I, what do you call it?
I would just call it dp 'cause I'm someone who abbreviates everything.
But you continually calling it Dynamics Profiler Dynamics.
Profiler Dynamics profiler.
Yeah. I have to,
that's part of my job to always have the full name and I cannot abbreviate. Um,
it, it hurts me. I can I say it here,
it hurts me when I see every scan with a big capital Ss written in the middle of
the name, which is, so it's, it's, it's one of the things that I am,
I'm always very much aware of because finding the right name is such a tedious
process and, um, when you have one, um, but still that's, uh, maybe just my,
my character. Um, we are, we are diverting from the actual topic. Yeah.
So don't, of
Course the question,
tell us what is different about it or what is it about to f c s that wasn't
previously. Okay. What, what, what? Come on. What, what's good about it?
What's good about it? Okay, so, um, actually we did what's good about it.
We did actually ask our colleagues and some, some users, uh, what,
what does, uh, what makes an f c s expert, right?
So we asked the question to figure out how to make it easy.
So one thing is that we asked all those question questions and we took the
points one by one and said, okay, so if you need this to be an expert,
how can we take this and make it easier for you?
And so we made it easy and therefore accessible. So that's, um, by a visit.
So we introduced a visit that makes sure everything is aligned,
everything is every, all your hardware is correct.
You cannot choose the wrong objective lens because you need a certain quality,
um, of optics. Um, you have, you can only choose the right laser lines.
You can, you cannot really do anything wrong in this regard.
And then when you start measuring, uh, you get a color code if you are,
um, counts per molecules, which is essentially your signal,
if that's good or not. So that's one step.
So you make it easy in terms of taking away all those steps.
So this is only the user interface, that's
The user interface software. So that's, yeah,
so that's one part that's really good. Um, I would say where, um,
you are guided and you can learn doing it. Um, and it's, it's, um,
it's not as full as the normal f c s with options.
So it's a little bit limited, but,
so you can be not intimidated and still do what you need to do
at the beginning, and you can always go and become an expert from that.
I would say that's one thing. Um, that was one real big focus and, um,
where we did our homework. And then the other part comes from the area scan,
um, design. So you have those 32 elements,
essentially you have 32 little pfs, um,
that are arranged in a way that we know with a,
with a spatial distribution that we know. So how the, um,
how then the signal is, um, collected.
We can make use of this in two different ways.
And one is that we get the spatial information for, um, additional measurements.
So we can see is, uh, we can't really,
sometimes we can see if a molecule goes from left to right, not all the time,
but we can see if there are borders, we can see, um,
we can measure, um, it was one measurement, the speed,
so maybe we come to this in more detail. So that's really cool.
And then what came a little bit as a,
something that I wanted so much for this and came what's then
kind of coming, oh, no, we can't do it. Or Yes, we can, or maybe not. Oh yes,
it, it does work. What's the intensity? And it was, um, the,
um, that was the 32 elements, uh, being used,
how we use it.
It's that you can really go into very bright samples and that is helpful because
you don't have to care anymore. You can go into the, um,
low expression levels for normal f c s. There is, the performance is the same,
at least in, in, uh, in the, in the time resolution that it offers.
But you can go very bright. So, um, so you could measure, um, uh,
just, um, solution of something, um,
of a d if needed. And, uh,
I think we did this for some of the examples actually,
which makes sense when we talk maybe about that functionality. Um,
so you don't have to worry anymore. So you can just do your comparisons. And,
um, we didn't find a sample that was too bright, let's say this way.
And that was really helpful. And maybe the last, sorry,
it's maybe a bit long answer. The last step was the, um, was, uh, what,
uh, Chris mentioned, uh, those, uh, calibrations. So we did, um,
we did a calibration in is,
so there are no values for this kind of calibration in the software.
And you can decide yourself because there's a certain amount of error from
system to system, not in between measurements, but from system to system.
So we give a kind of mean value and say, that's a, that will be good,
but your system could be plus minus some percent are different.
But then you can make the decision, is that good enough for me?
Do I just roughly compare something?
And then you could leave the calibrations off and still get your values,
or you can still do the calibration and put this value in wherever you like.
It's all in the visit.
So you can do this even in the visit and then get a a for your system or correct
answer, so to speak. Um, and compare better in between systems.
So if you're working in cooperation with somebody on a different site,
then those values are better comparable if you do this kind of calibration,
obviously, those kind of things. So it's lots of nitty gritty details, but then,
so it's more simple. Uh, you can essentially measure everything and yeah,
we come to the new information with the spatial air scan detector. I guess,
How long did it take to actually go from
concept of, of this is what you're going do to today,
where you've just launched a product around this.
How long did that process take?
It's longer than it was longer than a year. It's longer than a year,
maybe one and a half. Okay. From concept to product.
That's pretty fast. I, I,
in scientific world and the calibrations and all the testing and robustness,
I think that's pretty fast, actually. What, what's your ty what,
for most products, what's the typical timeline then?
Hmm. There is no typical timeline. Sorry.
There is just no typical,
and it would make our lives a lot easier if we could just say, oh,
this will always take such and such. But at the end, um,
you look at it and then you make a plan, and then you stick to the plan.
Best I best idea situation is to do that.
But things change, I guess. Just like any scientific experiment, you,
you start here to get here, but as you, as you go along, things crop up,
things change, and you have to change your path to get there, I guess. Mm-hmm.
That I, I'm gonna ask Austin, have you, uh,
how to play with Dynamics profiler?
Uh, yes. A little bit.
And how, how, what, what are your thoughts as someone who's, uh,
comes from a purist f c s background?
What is your thoughts on Dynamics Profiler? What do you like about it?
Maybe I should start there.
Yeah, no, what, what Annette already said. I mean, there's a couple of, of,
of nice features that keep you on track, that give you feedback. Um,
I think there's different ways to do that, but Dynamic Profiler is doing that,
actually, I'm, I'm sorry, um, is doing that actually, uh, quite well.
I think that should help actually a lot of users to get into this. Um,
in, in, okay, in our, let me do it slightly differently now, lab, we, we,
we just, we just sit somebody and, and,
and take a look at the correlation function because actually after you've done
two or three, you know, whether a correlation function is good or not,
you just don't know what it means yet. Mm-hmm. So, um,
we're trying to do this as well, very interactively.
And I think dynamic profile is doing this actually quite well. I think it's,
it's, it's much easier to use than,
Than, than than earlier systems. And Chris, so,
so you've come from a background of tests and a team help you do f c s.
You've sat down now and done this yourself in person. What, what are,
what is your impression? What are your thoughts?
I, yeah, so I came into it just, you know,
like quite happy to blindly test it. We did a very simple experiment where
we, we've previously defined different regions of the plasma membrane.
One that we, yeah, this is a good example. So it's a weird name.
The iso zone is this structure that's found in, in yeast,
and yet if Pete's ducking down,
you can see it's these little punctate dots about the PAs membrane,
especially when we focus on the top of the cell.
And that's different than other cargoes that might be more dispersed throughout.
So based on this knowledge and the fact that exosomes had been described as
static, but at least very low level, uh,
low levels of dynamics, we did that.
And another protein that we predicted would actually move very fast.
So something that's involved in
fusion of fast moving vesicles. So that was our,
our sledgehammer that should give us a delta, right? And then we,
we threw another thing in, an unknown in, and then again,
Pete's gonna have to duck 'cause I didn't format these for him to be standing in
front of him, but what you can see is on the right,
the things we predicted would be very low, uh,
with regards to the diffusion coefficient was indeed our fast thing was
very fast, and the unknown was slower than, you know,
somewhere in the middle. So that was pretty encouraging, you know,
just from taking something out the freezer to, to test and whether it, it fit.
And then I think the next day, I haven't sent the, the data,
but those fast moving things, I treated those cells with a compound to,
to essentially kill the cell or at least halt enzymatic processes.
And then I repeated the analysis and, you know, the, the dynamics were gone.
So as a, a, a very basic user,
it did appear to be reporting on the,
the physics of those molecules in a way that, that we expected.
But I, one other one other advantage actually.
So I put those in the freezer and then I think like a month later,
uh, a student I co supervised was actually doing a placement with Pete,
and she went on to do some dynamics profiling as part of that placement.
And if you look at her data and my data, they're remarkably similar.
So again, I was, you know, going into it with,
with nothing to no expectations,
but actually being genuinely quite impressed with the results we got.
I've gotta say, so from my side, so actually if,
if I'm gonna come into my own podcast now, which is a bit unusual from,
from the core facility perspective, you know, one of the ponds with f c s,
it's always needs one of, always needed one of my staff. So whether it be Grant,
Graham, Karen, Joe to be there with them, doing it for them,
or tests as a postdoc, uh, in, in Hitchcock's lab when she was there,
we needed that level of expertise to support the user.
It wasn't something we could easily let a user loose on very e very quickly,
because quite often they'd do a few experiments go away and it'd be months
before they came back to do the next set of experiments. By which point,
I think, unless you mentioned earlier, by the time you come back,
you've forgotten how to do your controls and everything else.
What was really good with this from my perspective,
is actually Chris could do it, Blythe could do it, uh, so the students, uh,
is referring to and they could do it really well and reliably.
And that was super cool. And maybe the,
the biggest credit to the user friendliness was that I could do it,
which is always a good test. Uh,
although my team are probably laughing if they're watching or listening to this
right now thinking, yes. So he thinks, uh, but actually I,
I recall, uh, my PhD student at Laura Wiggins,
we were doing some F frappe experiments. No, we weren't doing frap,
we were doing,
we were looking at some static situations and there was something odd about the
sample and we couldn't understand what was going on. So we thought, well,
actually we should wrap this. But actually frap,
this is the first time actually the dynamics profiler made doing f c s quicker
and easier to get more my result than Frap was.
And as a hardcore frap person, that that was quite exciting. It was like, oh no,
let's not use this. Ran next door, got it onto the beat test,
tried it with the F C s, and then we could try at the low expression levels,
medium expression levels, high expression levels,
we could see the concentration of the protein that we were interested in,
and we could see that the dynamics were constant.
So we weren't biasing it towards what our eyes were drawn to the prettier
pictures of the bright ones. But going right down to the low expression,
seeing that it was still relevant, uh,
for that data to be able to do that within half an hour
and then another two hours of repeats to, to make sure it is absolutely robust.
It's half an hour told us, we think we are honest,
but then obviously you have to repeat it and takes time to then do the repeat.
But it was super simple. As I say, it was me who was doing it. I,
Annette you were actually around at the time you were leaving at that moment.
Yeah, yeah, yeah. We were heading out.
So you were leaving out the door and I jumped in, grant gone with you,
which meant I was alone with Pete and having to do this, but it worked.
And that's what was so brilliant.
We could check that we weren't studying artifactual biology and we could
understand the basics of it. And so, back to you, Annette,
what was the biggest challenge in its development?
What was the biggest hurdle to overcome?
Oh, okay. What was the biggest hurdle to overcome?
When you're developing anything, you must get to a block a point,
just like when we're doing research,
you get to a point where you know where you want to go to,
but you just can't get over that hurdle for some reason.
What was the biggest barrier? What was, what slowed it down the most?
I think, um, I think there was not,
funnily enough, there was not a big block.
So the technology is very straightforward. The,
the vision was very straightforward. I think we did take an extra round,
really for the visit and,
or multiple extra rounds for the visit and the ease of use and with
alpha testing and beta testing,
and then making sure that simplifying it while not
cutting away too much of the possibilities, uh,
for applications that we might not even know yet.
So, because as I said, if you open a door, you might not know what you find.
That's the same for us, of course. And, um, I mentioned the, the,
the spatial measurements, the asymmetric, uh,
measurements that we do or the flow measurement, um, that we can do, you know,
that's s symmetric thank you,
where you can see differences in molecular behavior and characteristics in
one points per function. And the flow,
which gives you the option to something is moved actively, uh,
to determine speed and direction. So
not a lot of people have then, um, an experiment sitting on their shelf,
which we can try out.
So we had to really develop ourselves, uh,
tests and applications and look what works and what doesn't, which is again,
less straightforward than resolution, speed and sensitivity. Um,
fantastic features, but more straightforward in our world.
And so I guess that took us a little bit, uh, longer. Um,
but other than that, I
cannot say that there was a big block. I guess we,
um, we did, um, ask our, um,
developer or developers, but were in peculiar, um, many,
many times to explain stuff to us as we, as if we were five year olds,
like how, how this works, this asymmetry and this flow, because again,
we need to translate it right for everybody. So we would ask this question many,
many times. Um, and lucky for all of us, um,
our colleagues are extremely patient and very good at translating, so I'm,
uh, very grateful there. So yeah, that was very, that's a very fun project.
I, I, I think so, so,
so for those who are listening as an image on the visuals at the moment,
which actually looks a bit like a trivial pursuit Yes.
Game where there's two or two wedges to be one, uh, it's true.
And actually, I,
I find this part actually conceptually the hardest to understand how it works
because it depends on where you are, where you drop your spot.
If I can just go to another image, I dunno if this one will have it. It's, yeah,
that's,
So this is an
Image of a few cells and you've got, uh,
essentially to like targets. Uh,
so if you were a top pilot type thing, you've got your little cross hairs,
and that's where you're going to f c s and you've got multiples of these.
And of course the,
the dynamics in those regions will depend on the orientation of the,
the cell, the shape, everything else. So making sense of flow,
actually, I find conceptually, oh
Yeah, this is the asme. Yeah, but this is the asymmetric measurement and here,
so it's not about flow, what is happening here. So those are, um,
condensates and, um, oh, the reference is not all in there,
so I hope, um, I will be forgiven. Um, so this,
this are cellular condensates and what you see,
the little circles are actually the diameter of the ery scan detector.
So you always see where you measure exactly in your image.
It's not just a cross there, but you see exactly where your borders are.
And then on the right hand side in this image, you see, um,
a graphics with a heat map, so from red to blue, um,
displaying concentration and, um, movement. Um,
it doesn't tell you direction, it doesn't tell you speed. It's, um,
it's diffusion based, but you can see, um, you can really visualize differences.
And here those condensates dynamically form and then dynamically disperse
again. And, um, so f c s here, um,
so those are essentially two flies with one hit is that you can now, um,
go and do kind of normal F C Ss measurement, um,
but then characterize the borders with the asymetry. And, um,
those condensate get very, very bright because the,
the proteins assemble and concentrate and you couldn't measure
anything there. So, um, so those two, um,
components of the dynamics profile are not come together that you brightness is
not an issue and you have this opportunity, um, for the spatial thing.
There's another, um, example I for flow, uh,
the microfluidic system where, um, I sent you an image,
I hope I labeled it right, uh, where you yes,
where you can see this was a, so this is a microfluidic capillary system,
which we, uh, just filled with a solution.
And what we measured is, um, essentially a profile of the flow.
So you would get, um, those machines kind of, um, mean, um, value of, uh,
how much of your fluid would pass through your capillary. And, um,
now if you're really interested what yourself or your organoids or spirit
experience, um,
in terms of flow or force or kind of movement,
you can now measure this, um,
with the flow and there that gives you actually micrometers per second speed and
the correct direction. And, um, you can see how this, um, kind of,
depending on how far away you are from the capillary wall,
your speed is changing. So there, so there is a graph,
and surprisingly enough you need from this,
I think it's a 500 or a millimeter diameter,
I think it needs almost 200 microns to actually go to the full speed.
And then it's, um, uh, uh, homogeneous and then it goes down of course again.
And that's something that you would have maybe not known or guessed,
or this is now, it's now visible.
So, um, so this is, so this is more straightforward, I would say,
to understand flow, for me at least is more straightforward, but, um, complete,
it gives you completely different, um, set of information. So it's, yeah,
I, I, I, you know, it think I, I had until now, which is really stupid,
haven't been involved for so long to think about actually,
when we do have microfluidic devices,
we can actually test the speed that we're actually pumping it through to make
sure every time that we use it, uh,
which is not really interested in the biology,
it's just making sure the conditions we're studying,
the biology are consistent by just having a,
a fluorescence that's not gonna interfere just in solution to test the flow
rate. I, I also like the fact that, again,
the concentration can be tested to make sure the concentration is consistent,
right? I still haven't done it,
but I still want to put my antibodies on there after one month, six months,
and 12 months in storage and look at my antibody concentration and look to see
if we're getting change of aggregation states as well within it. Uh,
so again, it, it's kind of just a useful tool, uh,
that's not necessarily related to the biological, but it's just,
it has other, other functionalities that I think could be kind of,
maybe that's just a geek in me useful to, to use as a,
as a tool for other things other than just biological applications. Torsten.
Yeah. What, what are your, 'cause we've actually only got five minutes left.
Mm-hmm. So time has really flown.
What are your final thoughts around dynamics?
Profiler and I then have one more question for you after that.
So what are your thoughts around the dynamics profile at first and what are you
using it for?
Are you still using it in anger or are you still really great dynamics?
Profiler exists, I'm onto the next thing for f C Ss. Let,
let's look at the future. Where's it going?
So for us, I mean for us it's actually important for the, for the facility,
right? So we, we have, we have, uh, exactly the same issue actually.
People don't use actually f c s that incredibly often, uh,
they typically come to us and then they want us to do the measurements or so we
train the students or whatever. So it's dynamic profile that is of course,
much easier. They can actually go to a system, go there,
be guided and, and get the first results. So for us,
ourselves, uh, we, we go in a, in a, in a set slightly different direction.
We're trying to, uh,
look at things like artificial intelligence to help interpret the f c s data,
et cetera. And, uh, I, I, I,
I think ultimately that's one of the things that think gonna gonna go,
because that will help as well in, in, in interpreting data. But I,
I wanna make one point very quickly,
which to give you a little bit of a different, uh, uh, um, uh,
angle on, on, on f c s in principle,
if you do your measurements right, and the only thing you have to do, the, the,
the instrument always stays exactly the same.
You just have to measure a little bit faster. You still get your image,
you just get many, many images. I mean, you can compress, you can project them,
you get your normal image, but if you emission fast enough,
you can actually just get actually f c s along for free. The,
you know,
the systems we have now are almost all capable of doing ffc s in one way or
another. The only thing is, do you want to extract your information or not?
Why not? Because it basically comes along for free, right?
You just have to measure a little bit faster and perhaps,
and on corn forklifts is a little bit longer as well, but, you know,
So that's what we are looking towards, towards in the future as well
and making it even more widely applicable and always there. Uh,
my one last question was the maths that are involved in the analysis and the
predictive maths and the machine learning. I'm suspecting, in fact, you kind of,
what you just said kind of alluded a bit to this.
We can probably get even more from less in the way of data to understand our
samples going forward.
Some maths and machine learning help us predict and understand what's going on.
Do you see that being a big area of development over the next five to 10 years?
Oh yeah, absolutely. I, I, I think that this is what you said is exactly right.
You can get more from less because at the moment f c s measurements, you know,
you need, uh, several seconds, right? 10 seconds or whatever.
There was just, uh,
Hamm from Minnesota just actually published a very nice article where he shows
that he can actually get, uh, diffusion coefficient from shorter data. Um,
we just have, uh, uh, uh,
looked at at AI and trying to get as well actually data from much shorter, uh,
tracers. So you don't have to measure that long anymore.
So if you don't have to measure lung anymore, right?
It becomes more practical and then basically just get it along with imaging and,
uh, again, the mathematics, I think, uh, the, the moment you actually use ai,
the mathematics is not, it's not, it's not the issue anymore, actually.
Yeah,
I think so you
Don't need even correlation functions per se anymore.
You can directly take a look at the raw data, right? So, but, but this is,
I think where the whole thing's going. I think in the, in, in the long run,
actually super resolution, different single molecule techniques,
they're gonna come together because you can basically measure most of them
actually in, in, in, in, in a single measurement.
I think this is really exciting going forward. And Chris,
I'm gonna come to you as an end user, you know, where,
how do you see yourself using this and haven't just heard what Thorston said?
Come on, you must, you, I know you, you must be already thinking, oh my God,
I'm gonna now be looking at this, this, this.
How quickly can you develop that for us please?
So based on what Thorston just said, uh, one thing I tried,
and it's probably at the, the limit of the parameters.
We we're using the imaging time and, you know, things were getting bleached,
everything we image is in, uh, endosomal tag, um,
chromosomally tag. So it's endogenous levels.
Some of them in yeast might only be a few hundred molecules per cell,
so pretty low level.
So one thing I'd like to do is time-lapse f c s,
which could come with, you know, the, the shorter imaging, uh,
profile you said because yeah, I think we, we wouldn't be able to do it,
but it would be really nice to get some F C SS measurements,
change the environment off the same cell,
and then image again to get the ffc s because I think when you,
even with super resolution,
there could be massive changes in these dynamics that don't really look
different by confocal or super rats or whatever.
So I think getting your finger on the pulse of these biophysical changes that
can be very fast and very dramatic would, would be what,
what we'd be interested in using for sure.
And suppose high throughput, you know,
like if we could segment cells and use,
I'm not selling size instruments,
but the CD seven coupled with 80 scan could presumably do this at,
you know, high, high throughput, so you could screen libraries for
that, that kinda dynamic information,
Which comes back to the future as well.
And taking the whole images and being able to take big screens and looking at
subtle differences with even within the same,
within the same well that you can look at differences when it's internalized,
not internalized localization. Yeah.
And Annetta with you to say some final words and
go on. What have, what have I missed? What have I not asked you,
you wish I'd asked you? Is there anything,
Oh, what have I got? Um,
so I think, so anything you want to know about it, I think you can find online.
So I was, I will finish with not so much the,
something about the product or the, the dynamics profiler, but more that,
um, when you asked me about, um, the hurdles, um,
I think my final thought would be to really say that the best thing of this for
me, the best experiences, um, is this, uh,
dynamics profiler was to really see ourselves. So our colleagues in Yna, um,
and lots of people having fun at the system,
it's always fantastic if you come with something that you haven't done before
and people start having a lot of fun and ideas
and, um, having this very positive energy. And I think, um,
that what, that's what I will connect with this period, um, in,
in my life definitely quite a bit. And I don't know if you, um, I,
I will not say names,
but somebody in York was not an F C SS expert was ca came in and asked
me, so what does this curve? We said, that's a correlation.
And then we explain correlation and then two hours later, uh,
this person we were discussing about the correlation curve and this person would
kind of come up and say,
this is obviously a two component fit and this changed from,
I don't know what this curve is and this, and it was true, uh, to this,
this is obviously a two component it and having this very positive energy and
fun. I would say that's something that is, um,
that I do connect with Dynamics profile and I like to share. I think that's,
that's really what I like to share. Yeah. Uh,
And that, that, that's brilliant. Thank you. And I, I,
and actually thank you for all the developments from the end user perspective.
It makes a big difference to us. Yeah, we are hard, I'm a hardcore to,
you are a hardcore microscopist. We have users such as Chris,
who actually secretly a hardcore microscopy. He just hasn't completely, uh,
got the tattoo for it yet. Uh,
but it's making these technologies applicable,
which means people can ask address biological questions that they couldn't
otherwise easily address or would shy away from. It's opened a door,
which I think is used like going through a new frontiers.
It has opened the door and unlocked it for people to really see the potential
and understand their fundamental biology so much better,
which you have to understand the fundamentals to move on. Uh, so I, I chose you.
That is up to the hour, so I have to thank you all.
I should quickly point out though, don't forget, Petra,
one of the big stars of ffc s as well. It was a lovely podcast with her, uh,
a while ago in the series. So please go back to that and have a listen to that.
Thank everyone who's watched and listened,
but a big thank you to talk to Annette Chris. And do you know what?
I cannot wait to see the results that are coming outta it in the future.
Thank you very much. All thank you. Thanks a lot. Thanks.
Thank you, Pete.
Thank you for listening to the Microscopists,
a bite-sized bio podcast sponsored by Zeiss Microscopy.
To view all audio and video recordings from this series,
please visit bite-size bio.com/the-microscopists.
Creators and Guests
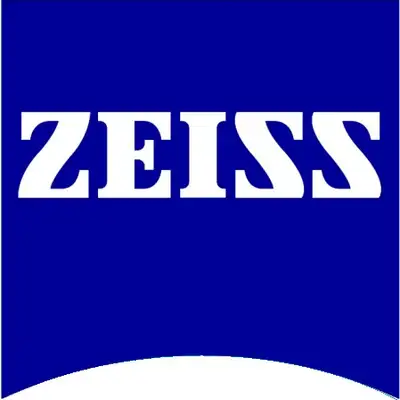
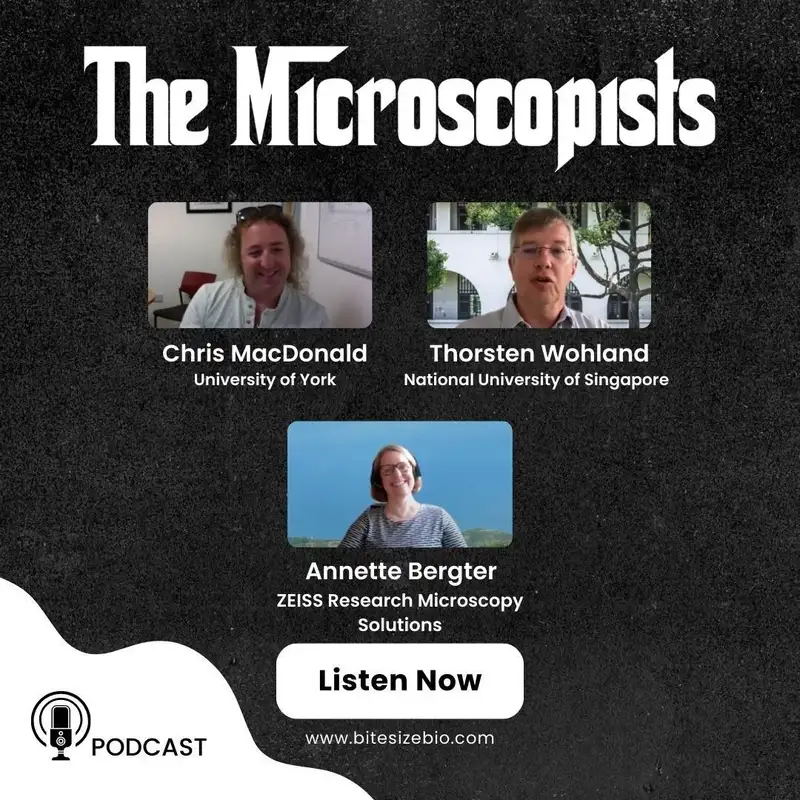